Covariance- v. correlation-matrix based PCA
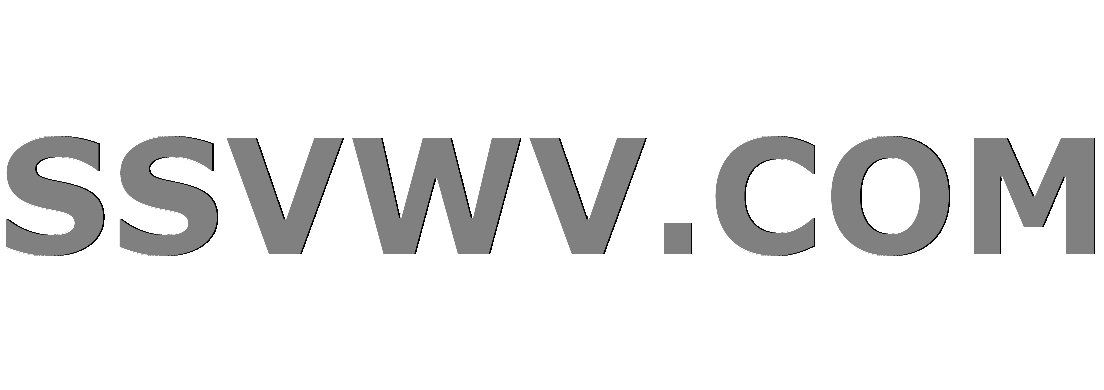
Multi tool use
up vote
5
down vote
favorite
In principal component analysis (PCA), one can choose either the covariance matrix or the correlation matrix to find the components. These give different results because, I suspect, the eigenvectors between both matrices are not equal. (Mathematically) similar matrices have the same eigenvalues, but not necessarily the same eigenvectors. Several questions: (1) Why this difference? (2) Does PCA make sense, if you can get two different answers? (3) Which of the two methods is 'best'? (4) Since PCA operates on standardized (not) raw data in both cases, i.e., scaled by their standard deviation, does it make sense to use the results to draw conclusions about the dominance of variation for the actual, unstandardized data?
linear-algebra statistics eigenvalues-eigenvectors
add a comment |
up vote
5
down vote
favorite
In principal component analysis (PCA), one can choose either the covariance matrix or the correlation matrix to find the components. These give different results because, I suspect, the eigenvectors between both matrices are not equal. (Mathematically) similar matrices have the same eigenvalues, but not necessarily the same eigenvectors. Several questions: (1) Why this difference? (2) Does PCA make sense, if you can get two different answers? (3) Which of the two methods is 'best'? (4) Since PCA operates on standardized (not) raw data in both cases, i.e., scaled by their standard deviation, does it make sense to use the results to draw conclusions about the dominance of variation for the actual, unstandardized data?
linear-algebra statistics eigenvalues-eigenvectors
If you scale them by their standard deviation, doesn't that make the covariance matrix into a correlation matrix?
– Michael Hardy
Jun 26 '13 at 13:09
This is more of a statistics question so is better asked at Cross Validated. You will probably get more/better answers there.
– kjetil b halvorsen
Jul 3 '14 at 9:14
add a comment |
up vote
5
down vote
favorite
up vote
5
down vote
favorite
In principal component analysis (PCA), one can choose either the covariance matrix or the correlation matrix to find the components. These give different results because, I suspect, the eigenvectors between both matrices are not equal. (Mathematically) similar matrices have the same eigenvalues, but not necessarily the same eigenvectors. Several questions: (1) Why this difference? (2) Does PCA make sense, if you can get two different answers? (3) Which of the two methods is 'best'? (4) Since PCA operates on standardized (not) raw data in both cases, i.e., scaled by their standard deviation, does it make sense to use the results to draw conclusions about the dominance of variation for the actual, unstandardized data?
linear-algebra statistics eigenvalues-eigenvectors
In principal component analysis (PCA), one can choose either the covariance matrix or the correlation matrix to find the components. These give different results because, I suspect, the eigenvectors between both matrices are not equal. (Mathematically) similar matrices have the same eigenvalues, but not necessarily the same eigenvectors. Several questions: (1) Why this difference? (2) Does PCA make sense, if you can get two different answers? (3) Which of the two methods is 'best'? (4) Since PCA operates on standardized (not) raw data in both cases, i.e., scaled by their standard deviation, does it make sense to use the results to draw conclusions about the dominance of variation for the actual, unstandardized data?
linear-algebra statistics eigenvalues-eigenvectors
linear-algebra statistics eigenvalues-eigenvectors
asked Jun 26 '13 at 13:00
Lucozade
60839
60839
If you scale them by their standard deviation, doesn't that make the covariance matrix into a correlation matrix?
– Michael Hardy
Jun 26 '13 at 13:09
This is more of a statistics question so is better asked at Cross Validated. You will probably get more/better answers there.
– kjetil b halvorsen
Jul 3 '14 at 9:14
add a comment |
If you scale them by their standard deviation, doesn't that make the covariance matrix into a correlation matrix?
– Michael Hardy
Jun 26 '13 at 13:09
This is more of a statistics question so is better asked at Cross Validated. You will probably get more/better answers there.
– kjetil b halvorsen
Jul 3 '14 at 9:14
If you scale them by their standard deviation, doesn't that make the covariance matrix into a correlation matrix?
– Michael Hardy
Jun 26 '13 at 13:09
If you scale them by their standard deviation, doesn't that make the covariance matrix into a correlation matrix?
– Michael Hardy
Jun 26 '13 at 13:09
This is more of a statistics question so is better asked at Cross Validated. You will probably get more/better answers there.
– kjetil b halvorsen
Jul 3 '14 at 9:14
This is more of a statistics question so is better asked at Cross Validated. You will probably get more/better answers there.
– kjetil b halvorsen
Jul 3 '14 at 9:14
add a comment |
1 Answer
1
active
oldest
votes
up vote
0
down vote
The problem with not standardizing, i.e. with not scaling the variables by their standard deviation, is that if, for example, one variable is measured in centimeters and another in dollars, then changing centimeters to meters can actually change the eigenvectors, so an arbitrary choice of units can alter the results. Hence I'd use the correlation matrix.
Correction to my part (4): "both cases" is incorrect; standardized variables are used in correlation-based PCA, not in covariance-based. But the issue and question still stands for the former.
– Lucozade
Jun 26 '13 at 13:23
Thanks Michael. Yes, this is the message/advice I am getting from literature too, but in case where the data are physically dimensionless, you still have a choice of two. It is not clear which one should be chosen on a more positive, fundamental basis.
– Lucozade
Jun 26 '13 at 13:29
My issue with scaling is that it seems to destroy the problem you are trying to solve. If you standardize each variable X by its own (= across different observations for the same variable) standard deviation, before performing correlation based PCA, how can it still make sense to look for directions of maximum variance for combinations of the variables, which is what PCA is all about? I know that that correlation based PCA is very convenient (standardized variables are dimensionless, so their linear combinations can be added; other advantages are also based on pragmatism), but is it correct?
– Lucozade
Jun 26 '13 at 23:04
It seems to me that covariance based PCA is the only truly correct one (even when the variances of the variables differ greatly) and that, whenever this version cannot be used, correlation based PCA should not be used either.
– Lucozade
Jun 26 '13 at 23:04
add a comment |
1 Answer
1
active
oldest
votes
1 Answer
1
active
oldest
votes
active
oldest
votes
active
oldest
votes
up vote
0
down vote
The problem with not standardizing, i.e. with not scaling the variables by their standard deviation, is that if, for example, one variable is measured in centimeters and another in dollars, then changing centimeters to meters can actually change the eigenvectors, so an arbitrary choice of units can alter the results. Hence I'd use the correlation matrix.
Correction to my part (4): "both cases" is incorrect; standardized variables are used in correlation-based PCA, not in covariance-based. But the issue and question still stands for the former.
– Lucozade
Jun 26 '13 at 13:23
Thanks Michael. Yes, this is the message/advice I am getting from literature too, but in case where the data are physically dimensionless, you still have a choice of two. It is not clear which one should be chosen on a more positive, fundamental basis.
– Lucozade
Jun 26 '13 at 13:29
My issue with scaling is that it seems to destroy the problem you are trying to solve. If you standardize each variable X by its own (= across different observations for the same variable) standard deviation, before performing correlation based PCA, how can it still make sense to look for directions of maximum variance for combinations of the variables, which is what PCA is all about? I know that that correlation based PCA is very convenient (standardized variables are dimensionless, so their linear combinations can be added; other advantages are also based on pragmatism), but is it correct?
– Lucozade
Jun 26 '13 at 23:04
It seems to me that covariance based PCA is the only truly correct one (even when the variances of the variables differ greatly) and that, whenever this version cannot be used, correlation based PCA should not be used either.
– Lucozade
Jun 26 '13 at 23:04
add a comment |
up vote
0
down vote
The problem with not standardizing, i.e. with not scaling the variables by their standard deviation, is that if, for example, one variable is measured in centimeters and another in dollars, then changing centimeters to meters can actually change the eigenvectors, so an arbitrary choice of units can alter the results. Hence I'd use the correlation matrix.
Correction to my part (4): "both cases" is incorrect; standardized variables are used in correlation-based PCA, not in covariance-based. But the issue and question still stands for the former.
– Lucozade
Jun 26 '13 at 13:23
Thanks Michael. Yes, this is the message/advice I am getting from literature too, but in case where the data are physically dimensionless, you still have a choice of two. It is not clear which one should be chosen on a more positive, fundamental basis.
– Lucozade
Jun 26 '13 at 13:29
My issue with scaling is that it seems to destroy the problem you are trying to solve. If you standardize each variable X by its own (= across different observations for the same variable) standard deviation, before performing correlation based PCA, how can it still make sense to look for directions of maximum variance for combinations of the variables, which is what PCA is all about? I know that that correlation based PCA is very convenient (standardized variables are dimensionless, so their linear combinations can be added; other advantages are also based on pragmatism), but is it correct?
– Lucozade
Jun 26 '13 at 23:04
It seems to me that covariance based PCA is the only truly correct one (even when the variances of the variables differ greatly) and that, whenever this version cannot be used, correlation based PCA should not be used either.
– Lucozade
Jun 26 '13 at 23:04
add a comment |
up vote
0
down vote
up vote
0
down vote
The problem with not standardizing, i.e. with not scaling the variables by their standard deviation, is that if, for example, one variable is measured in centimeters and another in dollars, then changing centimeters to meters can actually change the eigenvectors, so an arbitrary choice of units can alter the results. Hence I'd use the correlation matrix.
The problem with not standardizing, i.e. with not scaling the variables by their standard deviation, is that if, for example, one variable is measured in centimeters and another in dollars, then changing centimeters to meters can actually change the eigenvectors, so an arbitrary choice of units can alter the results. Hence I'd use the correlation matrix.
answered Jun 26 '13 at 13:16
Michael Hardy
1
1
Correction to my part (4): "both cases" is incorrect; standardized variables are used in correlation-based PCA, not in covariance-based. But the issue and question still stands for the former.
– Lucozade
Jun 26 '13 at 13:23
Thanks Michael. Yes, this is the message/advice I am getting from literature too, but in case where the data are physically dimensionless, you still have a choice of two. It is not clear which one should be chosen on a more positive, fundamental basis.
– Lucozade
Jun 26 '13 at 13:29
My issue with scaling is that it seems to destroy the problem you are trying to solve. If you standardize each variable X by its own (= across different observations for the same variable) standard deviation, before performing correlation based PCA, how can it still make sense to look for directions of maximum variance for combinations of the variables, which is what PCA is all about? I know that that correlation based PCA is very convenient (standardized variables are dimensionless, so their linear combinations can be added; other advantages are also based on pragmatism), but is it correct?
– Lucozade
Jun 26 '13 at 23:04
It seems to me that covariance based PCA is the only truly correct one (even when the variances of the variables differ greatly) and that, whenever this version cannot be used, correlation based PCA should not be used either.
– Lucozade
Jun 26 '13 at 23:04
add a comment |
Correction to my part (4): "both cases" is incorrect; standardized variables are used in correlation-based PCA, not in covariance-based. But the issue and question still stands for the former.
– Lucozade
Jun 26 '13 at 13:23
Thanks Michael. Yes, this is the message/advice I am getting from literature too, but in case where the data are physically dimensionless, you still have a choice of two. It is not clear which one should be chosen on a more positive, fundamental basis.
– Lucozade
Jun 26 '13 at 13:29
My issue with scaling is that it seems to destroy the problem you are trying to solve. If you standardize each variable X by its own (= across different observations for the same variable) standard deviation, before performing correlation based PCA, how can it still make sense to look for directions of maximum variance for combinations of the variables, which is what PCA is all about? I know that that correlation based PCA is very convenient (standardized variables are dimensionless, so their linear combinations can be added; other advantages are also based on pragmatism), but is it correct?
– Lucozade
Jun 26 '13 at 23:04
It seems to me that covariance based PCA is the only truly correct one (even when the variances of the variables differ greatly) and that, whenever this version cannot be used, correlation based PCA should not be used either.
– Lucozade
Jun 26 '13 at 23:04
Correction to my part (4): "both cases" is incorrect; standardized variables are used in correlation-based PCA, not in covariance-based. But the issue and question still stands for the former.
– Lucozade
Jun 26 '13 at 13:23
Correction to my part (4): "both cases" is incorrect; standardized variables are used in correlation-based PCA, not in covariance-based. But the issue and question still stands for the former.
– Lucozade
Jun 26 '13 at 13:23
Thanks Michael. Yes, this is the message/advice I am getting from literature too, but in case where the data are physically dimensionless, you still have a choice of two. It is not clear which one should be chosen on a more positive, fundamental basis.
– Lucozade
Jun 26 '13 at 13:29
Thanks Michael. Yes, this is the message/advice I am getting from literature too, but in case where the data are physically dimensionless, you still have a choice of two. It is not clear which one should be chosen on a more positive, fundamental basis.
– Lucozade
Jun 26 '13 at 13:29
My issue with scaling is that it seems to destroy the problem you are trying to solve. If you standardize each variable X by its own (= across different observations for the same variable) standard deviation, before performing correlation based PCA, how can it still make sense to look for directions of maximum variance for combinations of the variables, which is what PCA is all about? I know that that correlation based PCA is very convenient (standardized variables are dimensionless, so their linear combinations can be added; other advantages are also based on pragmatism), but is it correct?
– Lucozade
Jun 26 '13 at 23:04
My issue with scaling is that it seems to destroy the problem you are trying to solve. If you standardize each variable X by its own (= across different observations for the same variable) standard deviation, before performing correlation based PCA, how can it still make sense to look for directions of maximum variance for combinations of the variables, which is what PCA is all about? I know that that correlation based PCA is very convenient (standardized variables are dimensionless, so their linear combinations can be added; other advantages are also based on pragmatism), but is it correct?
– Lucozade
Jun 26 '13 at 23:04
It seems to me that covariance based PCA is the only truly correct one (even when the variances of the variables differ greatly) and that, whenever this version cannot be used, correlation based PCA should not be used either.
– Lucozade
Jun 26 '13 at 23:04
It seems to me that covariance based PCA is the only truly correct one (even when the variances of the variables differ greatly) and that, whenever this version cannot be used, correlation based PCA should not be used either.
– Lucozade
Jun 26 '13 at 23:04
add a comment |
Thanks for contributing an answer to Mathematics Stack Exchange!
- Please be sure to answer the question. Provide details and share your research!
But avoid …
- Asking for help, clarification, or responding to other answers.
- Making statements based on opinion; back them up with references or personal experience.
Use MathJax to format equations. MathJax reference.
To learn more, see our tips on writing great answers.
Some of your past answers have not been well-received, and you're in danger of being blocked from answering.
Please pay close attention to the following guidance:
- Please be sure to answer the question. Provide details and share your research!
But avoid …
- Asking for help, clarification, or responding to other answers.
- Making statements based on opinion; back them up with references or personal experience.
To learn more, see our tips on writing great answers.
Sign up or log in
StackExchange.ready(function () {
StackExchange.helpers.onClickDraftSave('#login-link');
});
Sign up using Google
Sign up using Facebook
Sign up using Email and Password
Post as a guest
Required, but never shown
StackExchange.ready(
function () {
StackExchange.openid.initPostLogin('.new-post-login', 'https%3a%2f%2fmath.stackexchange.com%2fquestions%2f429962%2fcovariance-v-correlation-matrix-based-pca%23new-answer', 'question_page');
}
);
Post as a guest
Required, but never shown
Sign up or log in
StackExchange.ready(function () {
StackExchange.helpers.onClickDraftSave('#login-link');
});
Sign up using Google
Sign up using Facebook
Sign up using Email and Password
Post as a guest
Required, but never shown
Sign up or log in
StackExchange.ready(function () {
StackExchange.helpers.onClickDraftSave('#login-link');
});
Sign up using Google
Sign up using Facebook
Sign up using Email and Password
Post as a guest
Required, but never shown
Sign up or log in
StackExchange.ready(function () {
StackExchange.helpers.onClickDraftSave('#login-link');
});
Sign up using Google
Sign up using Facebook
Sign up using Email and Password
Sign up using Google
Sign up using Facebook
Sign up using Email and Password
Post as a guest
Required, but never shown
Required, but never shown
Required, but never shown
Required, but never shown
Required, but never shown
Required, but never shown
Required, but never shown
Required, but never shown
Required, but never shown
f V5d6 4fUOTeM b0Csz,d0FBYOG,YWFNtOEVxyBJ2N4Foi24Ov7JfCM8xMIadC1cux F
If you scale them by their standard deviation, doesn't that make the covariance matrix into a correlation matrix?
– Michael Hardy
Jun 26 '13 at 13:09
This is more of a statistics question so is better asked at Cross Validated. You will probably get more/better answers there.
– kjetil b halvorsen
Jul 3 '14 at 9:14