Variance of the Euclidean norm under finite moment assumptions
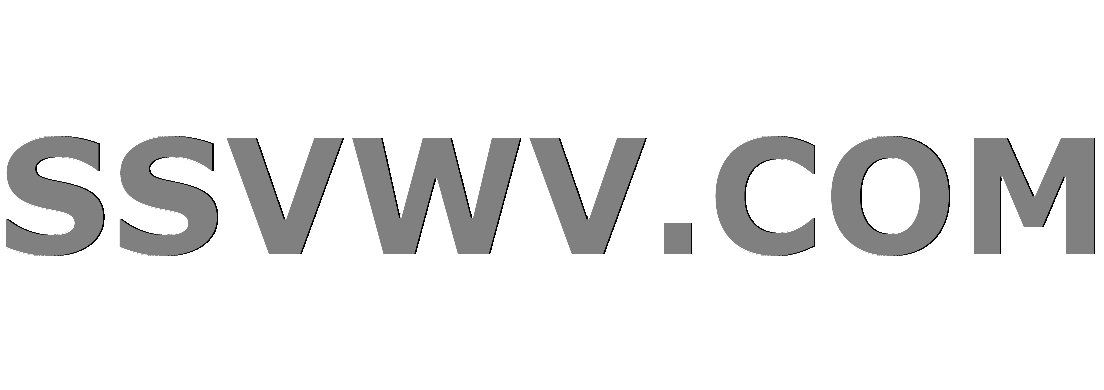
Multi tool use
up vote
4
down vote
favorite
Let $X = (X_1,X_2 cdots X_n)$ be random vector in $R^n$ with independent coordinate $X_i$ that satisfy $E[X_i^2]=1$ and $E[X_i^4] leq K^4$. Then show that $$operatorname{Var}(| X|_2) leq CK^4$$
where $C$ is a absolute constant and $| |_2$ denotes euclidian norm.
Here is my attempt:
$$begin{align*} E(|X|_2^2 -n)^2 &= E[(sum_{i=1}^n X_i^2)^2 ]-n^2 \
&=E[sum_{i=1}^n X_i^4]+E[sum_{i<j}X_i^2X_j^2] -n^2 \
&leq nK^4 + 2{{n}choose {2}}-n^2 \
&leq n(K^4-1) \
& leq nk^4
end{align*}$$
since $$ E(|X|_2^2 -n)^2 leq nk^4 rightarrow Eleft(frac{|X|_2^2}{n} -1right)^2 leq frac{K^4}{n}$$
and since
$$(forall z geq 0 |z-1|leq |z^2-1|) rightarrow
E(frac{|X|_2}{sqrt n} -1)^2leq E(frac{|X|_2^2}{n} -1)^2 $$
thus:
$$E(frac{|X|_2}{sqrt n} -1)^2 leq K^4/n rightarrow E(|X|_2-sqrt n)^2leq K^4$$
by Jensen inequality:
$$(E[|X|_2] - sqrt n)^2 leq K^4 $$
which is equivalence to
$$ |E[|X|_2] - sqrt n)| leq K^2$$
then when I am trying to bound $Var(| X|_2)$ I meet some problem :
$$operatorname{Var}(| X|_2)=E[|X|_2^2] -(E[|X|_2])^2 leq n- (K^2-sqrt n)^2 leq -K^4+2K^2sqrt n$$ which is not bound by constant , how can I bound that?
probability random-variables norm variance concentration-of-measure
add a comment |
up vote
4
down vote
favorite
Let $X = (X_1,X_2 cdots X_n)$ be random vector in $R^n$ with independent coordinate $X_i$ that satisfy $E[X_i^2]=1$ and $E[X_i^4] leq K^4$. Then show that $$operatorname{Var}(| X|_2) leq CK^4$$
where $C$ is a absolute constant and $| |_2$ denotes euclidian norm.
Here is my attempt:
$$begin{align*} E(|X|_2^2 -n)^2 &= E[(sum_{i=1}^n X_i^2)^2 ]-n^2 \
&=E[sum_{i=1}^n X_i^4]+E[sum_{i<j}X_i^2X_j^2] -n^2 \
&leq nK^4 + 2{{n}choose {2}}-n^2 \
&leq n(K^4-1) \
& leq nk^4
end{align*}$$
since $$ E(|X|_2^2 -n)^2 leq nk^4 rightarrow Eleft(frac{|X|_2^2}{n} -1right)^2 leq frac{K^4}{n}$$
and since
$$(forall z geq 0 |z-1|leq |z^2-1|) rightarrow
E(frac{|X|_2}{sqrt n} -1)^2leq E(frac{|X|_2^2}{n} -1)^2 $$
thus:
$$E(frac{|X|_2}{sqrt n} -1)^2 leq K^4/n rightarrow E(|X|_2-sqrt n)^2leq K^4$$
by Jensen inequality:
$$(E[|X|_2] - sqrt n)^2 leq K^4 $$
which is equivalence to
$$ |E[|X|_2] - sqrt n)| leq K^2$$
then when I am trying to bound $Var(| X|_2)$ I meet some problem :
$$operatorname{Var}(| X|_2)=E[|X|_2^2] -(E[|X|_2])^2 leq n- (K^2-sqrt n)^2 leq -K^4+2K^2sqrt n$$ which is not bound by constant , how can I bound that?
probability random-variables norm variance concentration-of-measure
Can you cite the source of your claim? My guess is that $text{Var}|X|$ will grow with $n$.
– nemo
yesterday
add a comment |
up vote
4
down vote
favorite
up vote
4
down vote
favorite
Let $X = (X_1,X_2 cdots X_n)$ be random vector in $R^n$ with independent coordinate $X_i$ that satisfy $E[X_i^2]=1$ and $E[X_i^4] leq K^4$. Then show that $$operatorname{Var}(| X|_2) leq CK^4$$
where $C$ is a absolute constant and $| |_2$ denotes euclidian norm.
Here is my attempt:
$$begin{align*} E(|X|_2^2 -n)^2 &= E[(sum_{i=1}^n X_i^2)^2 ]-n^2 \
&=E[sum_{i=1}^n X_i^4]+E[sum_{i<j}X_i^2X_j^2] -n^2 \
&leq nK^4 + 2{{n}choose {2}}-n^2 \
&leq n(K^4-1) \
& leq nk^4
end{align*}$$
since $$ E(|X|_2^2 -n)^2 leq nk^4 rightarrow Eleft(frac{|X|_2^2}{n} -1right)^2 leq frac{K^4}{n}$$
and since
$$(forall z geq 0 |z-1|leq |z^2-1|) rightarrow
E(frac{|X|_2}{sqrt n} -1)^2leq E(frac{|X|_2^2}{n} -1)^2 $$
thus:
$$E(frac{|X|_2}{sqrt n} -1)^2 leq K^4/n rightarrow E(|X|_2-sqrt n)^2leq K^4$$
by Jensen inequality:
$$(E[|X|_2] - sqrt n)^2 leq K^4 $$
which is equivalence to
$$ |E[|X|_2] - sqrt n)| leq K^2$$
then when I am trying to bound $Var(| X|_2)$ I meet some problem :
$$operatorname{Var}(| X|_2)=E[|X|_2^2] -(E[|X|_2])^2 leq n- (K^2-sqrt n)^2 leq -K^4+2K^2sqrt n$$ which is not bound by constant , how can I bound that?
probability random-variables norm variance concentration-of-measure
Let $X = (X_1,X_2 cdots X_n)$ be random vector in $R^n$ with independent coordinate $X_i$ that satisfy $E[X_i^2]=1$ and $E[X_i^4] leq K^4$. Then show that $$operatorname{Var}(| X|_2) leq CK^4$$
where $C$ is a absolute constant and $| |_2$ denotes euclidian norm.
Here is my attempt:
$$begin{align*} E(|X|_2^2 -n)^2 &= E[(sum_{i=1}^n X_i^2)^2 ]-n^2 \
&=E[sum_{i=1}^n X_i^4]+E[sum_{i<j}X_i^2X_j^2] -n^2 \
&leq nK^4 + 2{{n}choose {2}}-n^2 \
&leq n(K^4-1) \
& leq nk^4
end{align*}$$
since $$ E(|X|_2^2 -n)^2 leq nk^4 rightarrow Eleft(frac{|X|_2^2}{n} -1right)^2 leq frac{K^4}{n}$$
and since
$$(forall z geq 0 |z-1|leq |z^2-1|) rightarrow
E(frac{|X|_2}{sqrt n} -1)^2leq E(frac{|X|_2^2}{n} -1)^2 $$
thus:
$$E(frac{|X|_2}{sqrt n} -1)^2 leq K^4/n rightarrow E(|X|_2-sqrt n)^2leq K^4$$
by Jensen inequality:
$$(E[|X|_2] - sqrt n)^2 leq K^4 $$
which is equivalence to
$$ |E[|X|_2] - sqrt n)| leq K^2$$
then when I am trying to bound $Var(| X|_2)$ I meet some problem :
$$operatorname{Var}(| X|_2)=E[|X|_2^2] -(E[|X|_2])^2 leq n- (K^2-sqrt n)^2 leq -K^4+2K^2sqrt n$$ which is not bound by constant , how can I bound that?
probability random-variables norm variance concentration-of-measure
probability random-variables norm variance concentration-of-measure
edited Nov 26 at 14:29


Davide Giraudo
124k16150256
124k16150256
asked Nov 17 at 21:10


ShaoyuPei
1537
1537
Can you cite the source of your claim? My guess is that $text{Var}|X|$ will grow with $n$.
– nemo
yesterday
add a comment |
Can you cite the source of your claim? My guess is that $text{Var}|X|$ will grow with $n$.
– nemo
yesterday
Can you cite the source of your claim? My guess is that $text{Var}|X|$ will grow with $n$.
– nemo
yesterday
Can you cite the source of your claim? My guess is that $text{Var}|X|$ will grow with $n$.
– nemo
yesterday
add a comment |
active
oldest
votes
active
oldest
votes
active
oldest
votes
active
oldest
votes
active
oldest
votes
Thanks for contributing an answer to Mathematics Stack Exchange!
- Please be sure to answer the question. Provide details and share your research!
But avoid …
- Asking for help, clarification, or responding to other answers.
- Making statements based on opinion; back them up with references or personal experience.
Use MathJax to format equations. MathJax reference.
To learn more, see our tips on writing great answers.
Some of your past answers have not been well-received, and you're in danger of being blocked from answering.
Please pay close attention to the following guidance:
- Please be sure to answer the question. Provide details and share your research!
But avoid …
- Asking for help, clarification, or responding to other answers.
- Making statements based on opinion; back them up with references or personal experience.
To learn more, see our tips on writing great answers.
Sign up or log in
StackExchange.ready(function () {
StackExchange.helpers.onClickDraftSave('#login-link');
});
Sign up using Google
Sign up using Facebook
Sign up using Email and Password
Post as a guest
Required, but never shown
StackExchange.ready(
function () {
StackExchange.openid.initPostLogin('.new-post-login', 'https%3a%2f%2fmath.stackexchange.com%2fquestions%2f3002807%2fvariance-of-the-euclidean-norm-under-finite-moment-assumptions%23new-answer', 'question_page');
}
);
Post as a guest
Required, but never shown
Sign up or log in
StackExchange.ready(function () {
StackExchange.helpers.onClickDraftSave('#login-link');
});
Sign up using Google
Sign up using Facebook
Sign up using Email and Password
Post as a guest
Required, but never shown
Sign up or log in
StackExchange.ready(function () {
StackExchange.helpers.onClickDraftSave('#login-link');
});
Sign up using Google
Sign up using Facebook
Sign up using Email and Password
Post as a guest
Required, but never shown
Sign up or log in
StackExchange.ready(function () {
StackExchange.helpers.onClickDraftSave('#login-link');
});
Sign up using Google
Sign up using Facebook
Sign up using Email and Password
Sign up using Google
Sign up using Facebook
Sign up using Email and Password
Post as a guest
Required, but never shown
Required, but never shown
Required, but never shown
Required, but never shown
Required, but never shown
Required, but never shown
Required, but never shown
Required, but never shown
Required, but never shown
tB2WRICECXI8Q6zbb7pLr7 0RcFTBjtwpdMJB ECsfyNSeYjlUjL9 RDLW
Can you cite the source of your claim? My guess is that $text{Var}|X|$ will grow with $n$.
– nemo
yesterday