Why does changing random seeds alter results?
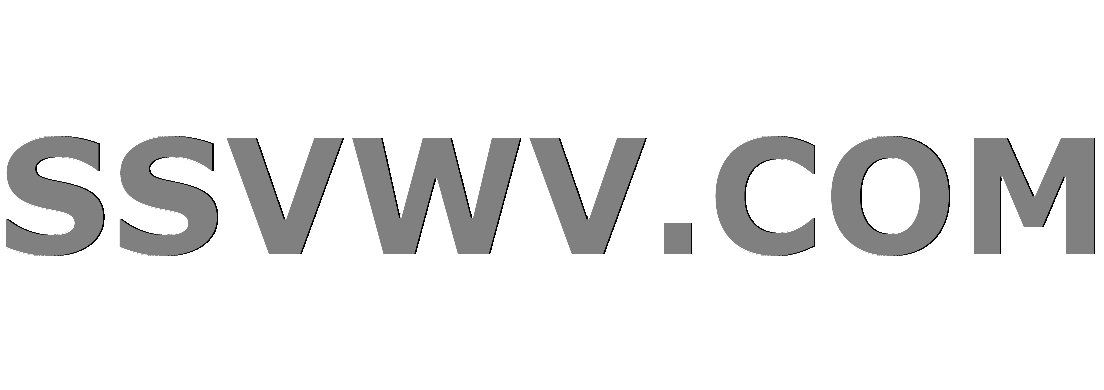
Multi tool use
.everyoneloves__top-leaderboard:empty,.everyoneloves__mid-leaderboard:empty{ margin-bottom:0;
}
up vote
5
down vote
favorite
I'm running some SVMs for a seminar and a friend of mine noted I should set a seed so my results don't change everytime I run the code. I was wondering why is that the case. If a different seed can induce different results, why should I trust SVMs at all?
Should I set a specific seed or is it ok to just set the first number that comes to my mind?
svm
add a comment |
up vote
5
down vote
favorite
I'm running some SVMs for a seminar and a friend of mine noted I should set a seed so my results don't change everytime I run the code. I was wondering why is that the case. If a different seed can induce different results, why should I trust SVMs at all?
Should I set a specific seed or is it ok to just set the first number that comes to my mind?
svm
5
"No man ever steps in the same river twice, for it's not the same river and he's not the same man." -- Heraclitus. Any time you do something, the results differ a little. Why should you demand, then, that a statistical procedure be any different? What matters isn't that the result changes, but by how much and whether it makes any difference. See stats.stackexchange.com/search?q=seed+set+random for discussions of this issue.
– whuber♦
Nov 18 at 19:45
add a comment |
up vote
5
down vote
favorite
up vote
5
down vote
favorite
I'm running some SVMs for a seminar and a friend of mine noted I should set a seed so my results don't change everytime I run the code. I was wondering why is that the case. If a different seed can induce different results, why should I trust SVMs at all?
Should I set a specific seed or is it ok to just set the first number that comes to my mind?
svm
I'm running some SVMs for a seminar and a friend of mine noted I should set a seed so my results don't change everytime I run the code. I was wondering why is that the case. If a different seed can induce different results, why should I trust SVMs at all?
Should I set a specific seed or is it ok to just set the first number that comes to my mind?
svm
svm
asked Nov 18 at 19:38
Pedro Cavalcante Oliveira
1534
1534
5
"No man ever steps in the same river twice, for it's not the same river and he's not the same man." -- Heraclitus. Any time you do something, the results differ a little. Why should you demand, then, that a statistical procedure be any different? What matters isn't that the result changes, but by how much and whether it makes any difference. See stats.stackexchange.com/search?q=seed+set+random for discussions of this issue.
– whuber♦
Nov 18 at 19:45
add a comment |
5
"No man ever steps in the same river twice, for it's not the same river and he's not the same man." -- Heraclitus. Any time you do something, the results differ a little. Why should you demand, then, that a statistical procedure be any different? What matters isn't that the result changes, but by how much and whether it makes any difference. See stats.stackexchange.com/search?q=seed+set+random for discussions of this issue.
– whuber♦
Nov 18 at 19:45
5
5
"No man ever steps in the same river twice, for it's not the same river and he's not the same man." -- Heraclitus. Any time you do something, the results differ a little. Why should you demand, then, that a statistical procedure be any different? What matters isn't that the result changes, but by how much and whether it makes any difference. See stats.stackexchange.com/search?q=seed+set+random for discussions of this issue.
– whuber♦
Nov 18 at 19:45
"No man ever steps in the same river twice, for it's not the same river and he's not the same man." -- Heraclitus. Any time you do something, the results differ a little. Why should you demand, then, that a statistical procedure be any different? What matters isn't that the result changes, but by how much and whether it makes any difference. See stats.stackexchange.com/search?q=seed+set+random for discussions of this issue.
– whuber♦
Nov 18 at 19:45
add a comment |
1 Answer
1
active
oldest
votes
up vote
12
down vote
accepted
tl;dr practically speaking, you can probably set the seed to anything you want (e.g. your birthday or phone number [although there are obvious privacy issues there :-)] or your lucky number); with some interesting caveats, you can use the same random number seed for most of your analyses (I often use 1001). In order to be useful, stochastic algorithms are generally insensitive to the random number seed.
the long answer
Classical statistical methods (t-test, ANOVA, regression etc.) are deterministic algorithms, but many modern algorithmic approaches include a stochastic component. (In between are methods like k-means clustering or expectation-maximization, which are intrinsically deterministic but are usually run from multiple randomly chosen starting points to mitigate their sensitivity to starting conditions.)
SVM need not be stochastic (e.g. the implementation in the e1071
package for R appears to be deterministic), but it is often implemented using stochastic gradient descent (SGD: e.g. see here) for computational reasons.
Methods that are using large ensembles of random samples from the data (e.g. bootstrapping, bagging, as well as SGD, which picks a different sample of the data at each update step) are effectively averaging across many samples, and are likely to be relatively insensitive to the random-number seed. Methods that are likely to be unstable with respect to the random-number seed (e.g. EM, k-means clustering) will generally have mechanisms built into the software that will automatically run several realizations and do something sensible with the results (i.e. average them), to make the method less sensitive.
This sensitivity is part of the information that you should know about a method before using it (along with some idea of its strengths and weaknesses, what meta-parameters it has that need to be tuned, etc.).
The best thing to do in the course of learning is to try some experiments - for a particular data set and model, try the same method with a handful of different random-number seeds and see how much the results vary!
+1. For a discussion of some of the issues associated with using arbitrary seeds, please see stats.stackexchange.com/questions/80407.
– whuber♦
Nov 18 at 21:35
add a comment |
1 Answer
1
active
oldest
votes
1 Answer
1
active
oldest
votes
active
oldest
votes
active
oldest
votes
up vote
12
down vote
accepted
tl;dr practically speaking, you can probably set the seed to anything you want (e.g. your birthday or phone number [although there are obvious privacy issues there :-)] or your lucky number); with some interesting caveats, you can use the same random number seed for most of your analyses (I often use 1001). In order to be useful, stochastic algorithms are generally insensitive to the random number seed.
the long answer
Classical statistical methods (t-test, ANOVA, regression etc.) are deterministic algorithms, but many modern algorithmic approaches include a stochastic component. (In between are methods like k-means clustering or expectation-maximization, which are intrinsically deterministic but are usually run from multiple randomly chosen starting points to mitigate their sensitivity to starting conditions.)
SVM need not be stochastic (e.g. the implementation in the e1071
package for R appears to be deterministic), but it is often implemented using stochastic gradient descent (SGD: e.g. see here) for computational reasons.
Methods that are using large ensembles of random samples from the data (e.g. bootstrapping, bagging, as well as SGD, which picks a different sample of the data at each update step) are effectively averaging across many samples, and are likely to be relatively insensitive to the random-number seed. Methods that are likely to be unstable with respect to the random-number seed (e.g. EM, k-means clustering) will generally have mechanisms built into the software that will automatically run several realizations and do something sensible with the results (i.e. average them), to make the method less sensitive.
This sensitivity is part of the information that you should know about a method before using it (along with some idea of its strengths and weaknesses, what meta-parameters it has that need to be tuned, etc.).
The best thing to do in the course of learning is to try some experiments - for a particular data set and model, try the same method with a handful of different random-number seeds and see how much the results vary!
+1. For a discussion of some of the issues associated with using arbitrary seeds, please see stats.stackexchange.com/questions/80407.
– whuber♦
Nov 18 at 21:35
add a comment |
up vote
12
down vote
accepted
tl;dr practically speaking, you can probably set the seed to anything you want (e.g. your birthday or phone number [although there are obvious privacy issues there :-)] or your lucky number); with some interesting caveats, you can use the same random number seed for most of your analyses (I often use 1001). In order to be useful, stochastic algorithms are generally insensitive to the random number seed.
the long answer
Classical statistical methods (t-test, ANOVA, regression etc.) are deterministic algorithms, but many modern algorithmic approaches include a stochastic component. (In between are methods like k-means clustering or expectation-maximization, which are intrinsically deterministic but are usually run from multiple randomly chosen starting points to mitigate their sensitivity to starting conditions.)
SVM need not be stochastic (e.g. the implementation in the e1071
package for R appears to be deterministic), but it is often implemented using stochastic gradient descent (SGD: e.g. see here) for computational reasons.
Methods that are using large ensembles of random samples from the data (e.g. bootstrapping, bagging, as well as SGD, which picks a different sample of the data at each update step) are effectively averaging across many samples, and are likely to be relatively insensitive to the random-number seed. Methods that are likely to be unstable with respect to the random-number seed (e.g. EM, k-means clustering) will generally have mechanisms built into the software that will automatically run several realizations and do something sensible with the results (i.e. average them), to make the method less sensitive.
This sensitivity is part of the information that you should know about a method before using it (along with some idea of its strengths and weaknesses, what meta-parameters it has that need to be tuned, etc.).
The best thing to do in the course of learning is to try some experiments - for a particular data set and model, try the same method with a handful of different random-number seeds and see how much the results vary!
+1. For a discussion of some of the issues associated with using arbitrary seeds, please see stats.stackexchange.com/questions/80407.
– whuber♦
Nov 18 at 21:35
add a comment |
up vote
12
down vote
accepted
up vote
12
down vote
accepted
tl;dr practically speaking, you can probably set the seed to anything you want (e.g. your birthday or phone number [although there are obvious privacy issues there :-)] or your lucky number); with some interesting caveats, you can use the same random number seed for most of your analyses (I often use 1001). In order to be useful, stochastic algorithms are generally insensitive to the random number seed.
the long answer
Classical statistical methods (t-test, ANOVA, regression etc.) are deterministic algorithms, but many modern algorithmic approaches include a stochastic component. (In between are methods like k-means clustering or expectation-maximization, which are intrinsically deterministic but are usually run from multiple randomly chosen starting points to mitigate their sensitivity to starting conditions.)
SVM need not be stochastic (e.g. the implementation in the e1071
package for R appears to be deterministic), but it is often implemented using stochastic gradient descent (SGD: e.g. see here) for computational reasons.
Methods that are using large ensembles of random samples from the data (e.g. bootstrapping, bagging, as well as SGD, which picks a different sample of the data at each update step) are effectively averaging across many samples, and are likely to be relatively insensitive to the random-number seed. Methods that are likely to be unstable with respect to the random-number seed (e.g. EM, k-means clustering) will generally have mechanisms built into the software that will automatically run several realizations and do something sensible with the results (i.e. average them), to make the method less sensitive.
This sensitivity is part of the information that you should know about a method before using it (along with some idea of its strengths and weaknesses, what meta-parameters it has that need to be tuned, etc.).
The best thing to do in the course of learning is to try some experiments - for a particular data set and model, try the same method with a handful of different random-number seeds and see how much the results vary!
tl;dr practically speaking, you can probably set the seed to anything you want (e.g. your birthday or phone number [although there are obvious privacy issues there :-)] or your lucky number); with some interesting caveats, you can use the same random number seed for most of your analyses (I often use 1001). In order to be useful, stochastic algorithms are generally insensitive to the random number seed.
the long answer
Classical statistical methods (t-test, ANOVA, regression etc.) are deterministic algorithms, but many modern algorithmic approaches include a stochastic component. (In between are methods like k-means clustering or expectation-maximization, which are intrinsically deterministic but are usually run from multiple randomly chosen starting points to mitigate their sensitivity to starting conditions.)
SVM need not be stochastic (e.g. the implementation in the e1071
package for R appears to be deterministic), but it is often implemented using stochastic gradient descent (SGD: e.g. see here) for computational reasons.
Methods that are using large ensembles of random samples from the data (e.g. bootstrapping, bagging, as well as SGD, which picks a different sample of the data at each update step) are effectively averaging across many samples, and are likely to be relatively insensitive to the random-number seed. Methods that are likely to be unstable with respect to the random-number seed (e.g. EM, k-means clustering) will generally have mechanisms built into the software that will automatically run several realizations and do something sensible with the results (i.e. average them), to make the method less sensitive.
This sensitivity is part of the information that you should know about a method before using it (along with some idea of its strengths and weaknesses, what meta-parameters it has that need to be tuned, etc.).
The best thing to do in the course of learning is to try some experiments - for a particular data set and model, try the same method with a handful of different random-number seeds and see how much the results vary!
edited Nov 18 at 22:38
answered Nov 18 at 21:05
Ben Bolker
21.7k15887
21.7k15887
+1. For a discussion of some of the issues associated with using arbitrary seeds, please see stats.stackexchange.com/questions/80407.
– whuber♦
Nov 18 at 21:35
add a comment |
+1. For a discussion of some of the issues associated with using arbitrary seeds, please see stats.stackexchange.com/questions/80407.
– whuber♦
Nov 18 at 21:35
+1. For a discussion of some of the issues associated with using arbitrary seeds, please see stats.stackexchange.com/questions/80407.
– whuber♦
Nov 18 at 21:35
+1. For a discussion of some of the issues associated with using arbitrary seeds, please see stats.stackexchange.com/questions/80407.
– whuber♦
Nov 18 at 21:35
add a comment |
Sign up or log in
StackExchange.ready(function () {
StackExchange.helpers.onClickDraftSave('#login-link');
});
Sign up using Google
Sign up using Facebook
Sign up using Email and Password
Post as a guest
Required, but never shown
StackExchange.ready(
function () {
StackExchange.openid.initPostLogin('.new-post-login', 'https%3a%2f%2fstats.stackexchange.com%2fquestions%2f377658%2fwhy-does-changing-random-seeds-alter-results%23new-answer', 'question_page');
}
);
Post as a guest
Required, but never shown
Sign up or log in
StackExchange.ready(function () {
StackExchange.helpers.onClickDraftSave('#login-link');
});
Sign up using Google
Sign up using Facebook
Sign up using Email and Password
Post as a guest
Required, but never shown
Sign up or log in
StackExchange.ready(function () {
StackExchange.helpers.onClickDraftSave('#login-link');
});
Sign up using Google
Sign up using Facebook
Sign up using Email and Password
Post as a guest
Required, but never shown
Sign up or log in
StackExchange.ready(function () {
StackExchange.helpers.onClickDraftSave('#login-link');
});
Sign up using Google
Sign up using Facebook
Sign up using Email and Password
Sign up using Google
Sign up using Facebook
Sign up using Email and Password
Post as a guest
Required, but never shown
Required, but never shown
Required, but never shown
Required, but never shown
Required, but never shown
Required, but never shown
Required, but never shown
Required, but never shown
Required, but never shown
FtU2SY2 4,7z ZBIT1I9UAwTg yYo4TQ,lB jo5Jy69318b4OSOyN4wBB7Y7M0eFGlU,vB
5
"No man ever steps in the same river twice, for it's not the same river and he's not the same man." -- Heraclitus. Any time you do something, the results differ a little. Why should you demand, then, that a statistical procedure be any different? What matters isn't that the result changes, but by how much and whether it makes any difference. See stats.stackexchange.com/search?q=seed+set+random for discussions of this issue.
– whuber♦
Nov 18 at 19:45