what is the best (one with the tightest bounds) concentration inequality for a continuous random variable...
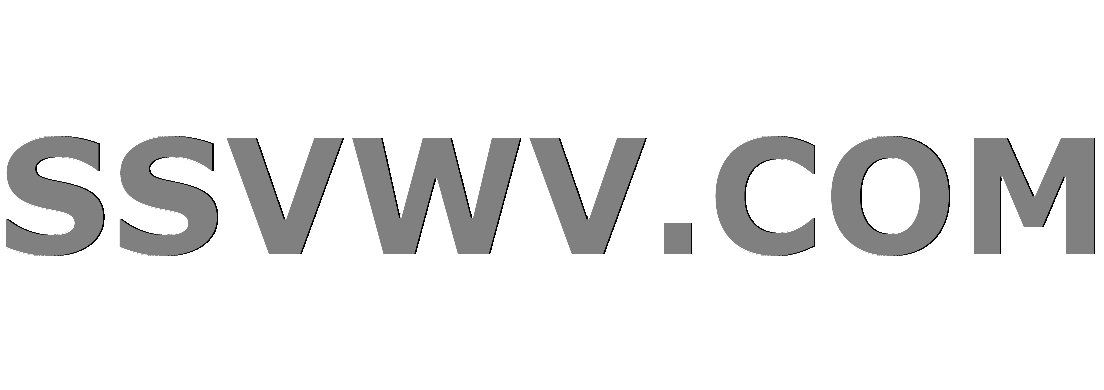
Multi tool use
up vote
0
down vote
favorite
what is the best (one with the tightest bounds) concentration inequality for a continuous random variable whose mean and max value is given? The random variable can take negative values.
probability statistics random-variables
New contributor
Larik is a new contributor to this site. Take care in asking for clarification, commenting, and answering.
Check out our Code of Conduct.
|
show 1 more comment
up vote
0
down vote
favorite
what is the best (one with the tightest bounds) concentration inequality for a continuous random variable whose mean and max value is given? The random variable can take negative values.
probability statistics random-variables
New contributor
Larik is a new contributor to this site. Take care in asking for clarification, commenting, and answering.
Check out our Code of Conduct.
Even if you're also given the minimum, I'm not sure you can actually do any better than Chebyshev's inequality, because the standard example for which Chebyshev is tight can be arbitrarily well approximated by continuous random variables. (Unless "concentration inequality" means something different than I think it means. I assume it means $P(|X-mu|>epsilon)<delta$.)
– Ian
Nov 15 at 14:37
If the minimum was given I would know the maximum value of the variance and chebyshev would become applicable. yes, thats what i mean by concentration inequality.
– Larik
Nov 15 at 14:46
So you're going for something weaker than Chebyshev? Chebyshev is already generally regarded as uselessly weak.
– Ian
Nov 15 at 14:47
No, chebyshev is good enough. I cant figure out how to actually apply it to this problem since I am not sure about the maximum value the variance can take
– Larik
Nov 15 at 14:52
You wind up needing something weaker than Chebyshev because the variance in this situation can be arbitrarily large. In the discrete setting, you can choose a variable with mean zero which is always equal to either $-M,0$ or $1$. This means $P(X=-M)=p/M,P(X=1)=p,P(X=0)=1-p(1+1/M)$ where $p$ is the one remaining free parameter and can be used to tune $sigma/M$. In the continuous setting you need to smear it out but you can do arbitrarily little smearing to obtain essentially the same result.
– Ian
Nov 15 at 15:00
|
show 1 more comment
up vote
0
down vote
favorite
up vote
0
down vote
favorite
what is the best (one with the tightest bounds) concentration inequality for a continuous random variable whose mean and max value is given? The random variable can take negative values.
probability statistics random-variables
New contributor
Larik is a new contributor to this site. Take care in asking for clarification, commenting, and answering.
Check out our Code of Conduct.
what is the best (one with the tightest bounds) concentration inequality for a continuous random variable whose mean and max value is given? The random variable can take negative values.
probability statistics random-variables
probability statistics random-variables
New contributor
Larik is a new contributor to this site. Take care in asking for clarification, commenting, and answering.
Check out our Code of Conduct.
New contributor
Larik is a new contributor to this site. Take care in asking for clarification, commenting, and answering.
Check out our Code of Conduct.
edited Nov 15 at 14:38
New contributor
Larik is a new contributor to this site. Take care in asking for clarification, commenting, and answering.
Check out our Code of Conduct.
asked Nov 15 at 14:35
Larik
11
11
New contributor
Larik is a new contributor to this site. Take care in asking for clarification, commenting, and answering.
Check out our Code of Conduct.
New contributor
Larik is a new contributor to this site. Take care in asking for clarification, commenting, and answering.
Check out our Code of Conduct.
Larik is a new contributor to this site. Take care in asking for clarification, commenting, and answering.
Check out our Code of Conduct.
Even if you're also given the minimum, I'm not sure you can actually do any better than Chebyshev's inequality, because the standard example for which Chebyshev is tight can be arbitrarily well approximated by continuous random variables. (Unless "concentration inequality" means something different than I think it means. I assume it means $P(|X-mu|>epsilon)<delta$.)
– Ian
Nov 15 at 14:37
If the minimum was given I would know the maximum value of the variance and chebyshev would become applicable. yes, thats what i mean by concentration inequality.
– Larik
Nov 15 at 14:46
So you're going for something weaker than Chebyshev? Chebyshev is already generally regarded as uselessly weak.
– Ian
Nov 15 at 14:47
No, chebyshev is good enough. I cant figure out how to actually apply it to this problem since I am not sure about the maximum value the variance can take
– Larik
Nov 15 at 14:52
You wind up needing something weaker than Chebyshev because the variance in this situation can be arbitrarily large. In the discrete setting, you can choose a variable with mean zero which is always equal to either $-M,0$ or $1$. This means $P(X=-M)=p/M,P(X=1)=p,P(X=0)=1-p(1+1/M)$ where $p$ is the one remaining free parameter and can be used to tune $sigma/M$. In the continuous setting you need to smear it out but you can do arbitrarily little smearing to obtain essentially the same result.
– Ian
Nov 15 at 15:00
|
show 1 more comment
Even if you're also given the minimum, I'm not sure you can actually do any better than Chebyshev's inequality, because the standard example for which Chebyshev is tight can be arbitrarily well approximated by continuous random variables. (Unless "concentration inequality" means something different than I think it means. I assume it means $P(|X-mu|>epsilon)<delta$.)
– Ian
Nov 15 at 14:37
If the minimum was given I would know the maximum value of the variance and chebyshev would become applicable. yes, thats what i mean by concentration inequality.
– Larik
Nov 15 at 14:46
So you're going for something weaker than Chebyshev? Chebyshev is already generally regarded as uselessly weak.
– Ian
Nov 15 at 14:47
No, chebyshev is good enough. I cant figure out how to actually apply it to this problem since I am not sure about the maximum value the variance can take
– Larik
Nov 15 at 14:52
You wind up needing something weaker than Chebyshev because the variance in this situation can be arbitrarily large. In the discrete setting, you can choose a variable with mean zero which is always equal to either $-M,0$ or $1$. This means $P(X=-M)=p/M,P(X=1)=p,P(X=0)=1-p(1+1/M)$ where $p$ is the one remaining free parameter and can be used to tune $sigma/M$. In the continuous setting you need to smear it out but you can do arbitrarily little smearing to obtain essentially the same result.
– Ian
Nov 15 at 15:00
Even if you're also given the minimum, I'm not sure you can actually do any better than Chebyshev's inequality, because the standard example for which Chebyshev is tight can be arbitrarily well approximated by continuous random variables. (Unless "concentration inequality" means something different than I think it means. I assume it means $P(|X-mu|>epsilon)<delta$.)
– Ian
Nov 15 at 14:37
Even if you're also given the minimum, I'm not sure you can actually do any better than Chebyshev's inequality, because the standard example for which Chebyshev is tight can be arbitrarily well approximated by continuous random variables. (Unless "concentration inequality" means something different than I think it means. I assume it means $P(|X-mu|>epsilon)<delta$.)
– Ian
Nov 15 at 14:37
If the minimum was given I would know the maximum value of the variance and chebyshev would become applicable. yes, thats what i mean by concentration inequality.
– Larik
Nov 15 at 14:46
If the minimum was given I would know the maximum value of the variance and chebyshev would become applicable. yes, thats what i mean by concentration inequality.
– Larik
Nov 15 at 14:46
So you're going for something weaker than Chebyshev? Chebyshev is already generally regarded as uselessly weak.
– Ian
Nov 15 at 14:47
So you're going for something weaker than Chebyshev? Chebyshev is already generally regarded as uselessly weak.
– Ian
Nov 15 at 14:47
No, chebyshev is good enough. I cant figure out how to actually apply it to this problem since I am not sure about the maximum value the variance can take
– Larik
Nov 15 at 14:52
No, chebyshev is good enough. I cant figure out how to actually apply it to this problem since I am not sure about the maximum value the variance can take
– Larik
Nov 15 at 14:52
You wind up needing something weaker than Chebyshev because the variance in this situation can be arbitrarily large. In the discrete setting, you can choose a variable with mean zero which is always equal to either $-M,0$ or $1$. This means $P(X=-M)=p/M,P(X=1)=p,P(X=0)=1-p(1+1/M)$ where $p$ is the one remaining free parameter and can be used to tune $sigma/M$. In the continuous setting you need to smear it out but you can do arbitrarily little smearing to obtain essentially the same result.
– Ian
Nov 15 at 15:00
You wind up needing something weaker than Chebyshev because the variance in this situation can be arbitrarily large. In the discrete setting, you can choose a variable with mean zero which is always equal to either $-M,0$ or $1$. This means $P(X=-M)=p/M,P(X=1)=p,P(X=0)=1-p(1+1/M)$ where $p$ is the one remaining free parameter and can be used to tune $sigma/M$. In the continuous setting you need to smear it out but you can do arbitrarily little smearing to obtain essentially the same result.
– Ian
Nov 15 at 15:00
|
show 1 more comment
1 Answer
1
active
oldest
votes
up vote
0
down vote
By scaling and translation, one can assume that the mean is zero and the max is $1$ (unless the distribution is degenerate). Having done so, splitting the variable into positive and negative parts allows you to apply Markov's inequality to see $P(X leq -a) leq frac{P(X>0)}{a}$ for $a>0$ (since the negative part of $X$ has expectation at most $P(X>0)$.) This then translates into a bound on $P(|X| geq a)$ once $a>1$. You can then undo the scaling and translation to return to the general case.
Is that the best you can do? Yes: one can look at a variable which is $-a$ with probability $1/a$ and $1$ with probability $1-1/a$ to see that. The continuous aspect does not diminish this, since one can mollify this example arbitrarily little to obtain a continuous example with arbitrarily similar properties.
add a comment |
1 Answer
1
active
oldest
votes
1 Answer
1
active
oldest
votes
active
oldest
votes
active
oldest
votes
up vote
0
down vote
By scaling and translation, one can assume that the mean is zero and the max is $1$ (unless the distribution is degenerate). Having done so, splitting the variable into positive and negative parts allows you to apply Markov's inequality to see $P(X leq -a) leq frac{P(X>0)}{a}$ for $a>0$ (since the negative part of $X$ has expectation at most $P(X>0)$.) This then translates into a bound on $P(|X| geq a)$ once $a>1$. You can then undo the scaling and translation to return to the general case.
Is that the best you can do? Yes: one can look at a variable which is $-a$ with probability $1/a$ and $1$ with probability $1-1/a$ to see that. The continuous aspect does not diminish this, since one can mollify this example arbitrarily little to obtain a continuous example with arbitrarily similar properties.
add a comment |
up vote
0
down vote
By scaling and translation, one can assume that the mean is zero and the max is $1$ (unless the distribution is degenerate). Having done so, splitting the variable into positive and negative parts allows you to apply Markov's inequality to see $P(X leq -a) leq frac{P(X>0)}{a}$ for $a>0$ (since the negative part of $X$ has expectation at most $P(X>0)$.) This then translates into a bound on $P(|X| geq a)$ once $a>1$. You can then undo the scaling and translation to return to the general case.
Is that the best you can do? Yes: one can look at a variable which is $-a$ with probability $1/a$ and $1$ with probability $1-1/a$ to see that. The continuous aspect does not diminish this, since one can mollify this example arbitrarily little to obtain a continuous example with arbitrarily similar properties.
add a comment |
up vote
0
down vote
up vote
0
down vote
By scaling and translation, one can assume that the mean is zero and the max is $1$ (unless the distribution is degenerate). Having done so, splitting the variable into positive and negative parts allows you to apply Markov's inequality to see $P(X leq -a) leq frac{P(X>0)}{a}$ for $a>0$ (since the negative part of $X$ has expectation at most $P(X>0)$.) This then translates into a bound on $P(|X| geq a)$ once $a>1$. You can then undo the scaling and translation to return to the general case.
Is that the best you can do? Yes: one can look at a variable which is $-a$ with probability $1/a$ and $1$ with probability $1-1/a$ to see that. The continuous aspect does not diminish this, since one can mollify this example arbitrarily little to obtain a continuous example with arbitrarily similar properties.
By scaling and translation, one can assume that the mean is zero and the max is $1$ (unless the distribution is degenerate). Having done so, splitting the variable into positive and negative parts allows you to apply Markov's inequality to see $P(X leq -a) leq frac{P(X>0)}{a}$ for $a>0$ (since the negative part of $X$ has expectation at most $P(X>0)$.) This then translates into a bound on $P(|X| geq a)$ once $a>1$. You can then undo the scaling and translation to return to the general case.
Is that the best you can do? Yes: one can look at a variable which is $-a$ with probability $1/a$ and $1$ with probability $1-1/a$ to see that. The continuous aspect does not diminish this, since one can mollify this example arbitrarily little to obtain a continuous example with arbitrarily similar properties.
answered Nov 15 at 16:27
Ian
66.8k24984
66.8k24984
add a comment |
add a comment |
Larik is a new contributor. Be nice, and check out our Code of Conduct.
Larik is a new contributor. Be nice, and check out our Code of Conduct.
Larik is a new contributor. Be nice, and check out our Code of Conduct.
Larik is a new contributor. Be nice, and check out our Code of Conduct.
Sign up or log in
StackExchange.ready(function () {
StackExchange.helpers.onClickDraftSave('#login-link');
});
Sign up using Google
Sign up using Facebook
Sign up using Email and Password
Post as a guest
Required, but never shown
StackExchange.ready(
function () {
StackExchange.openid.initPostLogin('.new-post-login', 'https%3a%2f%2fmath.stackexchange.com%2fquestions%2f2999779%2fwhat-is-the-best-one-with-the-tightest-bounds-concentration-inequality-for-a-c%23new-answer', 'question_page');
}
);
Post as a guest
Required, but never shown
Sign up or log in
StackExchange.ready(function () {
StackExchange.helpers.onClickDraftSave('#login-link');
});
Sign up using Google
Sign up using Facebook
Sign up using Email and Password
Post as a guest
Required, but never shown
Sign up or log in
StackExchange.ready(function () {
StackExchange.helpers.onClickDraftSave('#login-link');
});
Sign up using Google
Sign up using Facebook
Sign up using Email and Password
Post as a guest
Required, but never shown
Sign up or log in
StackExchange.ready(function () {
StackExchange.helpers.onClickDraftSave('#login-link');
});
Sign up using Google
Sign up using Facebook
Sign up using Email and Password
Sign up using Google
Sign up using Facebook
Sign up using Email and Password
Post as a guest
Required, but never shown
Required, but never shown
Required, but never shown
Required, but never shown
Required, but never shown
Required, but never shown
Required, but never shown
Required, but never shown
Required, but never shown
dpGxP QqfdhPVK,areEPGI9 E
Even if you're also given the minimum, I'm not sure you can actually do any better than Chebyshev's inequality, because the standard example for which Chebyshev is tight can be arbitrarily well approximated by continuous random variables. (Unless "concentration inequality" means something different than I think it means. I assume it means $P(|X-mu|>epsilon)<delta$.)
– Ian
Nov 15 at 14:37
If the minimum was given I would know the maximum value of the variance and chebyshev would become applicable. yes, thats what i mean by concentration inequality.
– Larik
Nov 15 at 14:46
So you're going for something weaker than Chebyshev? Chebyshev is already generally regarded as uselessly weak.
– Ian
Nov 15 at 14:47
No, chebyshev is good enough. I cant figure out how to actually apply it to this problem since I am not sure about the maximum value the variance can take
– Larik
Nov 15 at 14:52
You wind up needing something weaker than Chebyshev because the variance in this situation can be arbitrarily large. In the discrete setting, you can choose a variable with mean zero which is always equal to either $-M,0$ or $1$. This means $P(X=-M)=p/M,P(X=1)=p,P(X=0)=1-p(1+1/M)$ where $p$ is the one remaining free parameter and can be used to tune $sigma/M$. In the continuous setting you need to smear it out but you can do arbitrarily little smearing to obtain essentially the same result.
– Ian
Nov 15 at 15:00